Conclusion

Dr. Zach Solan
Ai Advisor
In the realm of data and assessments, reliability is a fundamental concept that researchers and analysts prioritize. It refers to the consistency of a measurement—if you assess something multiple times, the results should be the same each time. Think of an archer consistently hitting the same spot on a target—that’s reliability in action. Unreliable data can lead to incorrect conclusions and poor decisions, which is why researchers go to great lengths to ensure their measurements are both dependable and accurate. For example, Target A illustrates poor reliability, whereas Targets B and C demonstrate high reliability, clearly highlighting the crucial role reliability plays in the validity of data analysis.

Figure 1: What is reliability and validity?
In a classic scenario, two or more raters rank or score different candidates, as shown in Table 1. Imagine two raters, Emma and John, who are independently asked to evaluate drawings by 4-year-old children. Each child was instructed to draw a specific set of animals in a predefined order (e.g., cat, dog, cow, elephant).
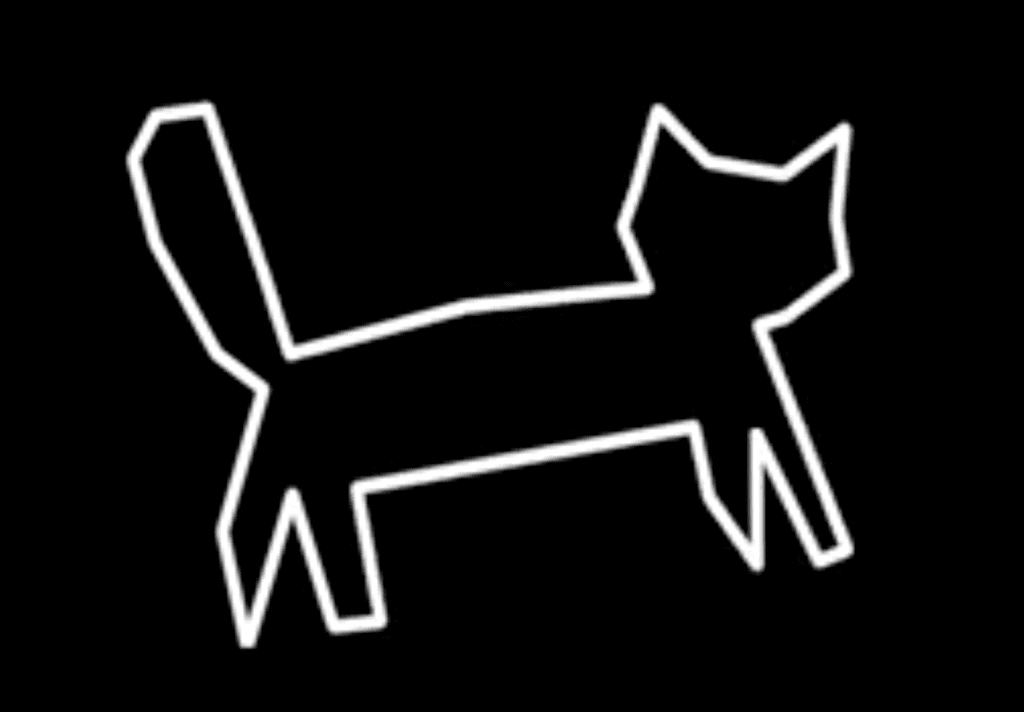
Can that be recognized as a cat?”
The raters are then required to indicate for each drawing whether the animal is recognizable (answering with a 1) or not (answering with a 0). Emma might believe the drawing resembles a cat and mark a 1, while John might see something different and mark a 0. The question is, how do we measure the reliability of this process?
This is a classic scenario for applying Cohen’s Kappa. The following video explains exactly how it is calculated:
Nevertheless, how do we calculate reliability in a scenario where two raters independently assess only a specific subject? For example, consider two parents who assess only their child’s behavior. The clinician who provides the assessment then receive these assessments—what happens next? How many of their answers should the clinician expect to Additionally, as shown in Table 2, the table has been modified to reflect the new group settings.
Traditionally, statisticians have used Cohen’s Kappa to measure the reliability of assessments, such as those conducted by parents. This method involves comparing the observed agreement between raters with the agreement we would expect if they were simply guessing. However, Cohen’s Kappa assumes that all items in the assessment have the same level of observed agreement—in our example, it assumes all drawings have the same probability of being correctly identified. But this isn’t always the case. For instance, a drawing of a cat is typically easier for a young child to identify than a drawing of a cow.
Moreover, we might want to compare Emma and John’s assessments with those of other parents evaluating the same drawings. But what if the children are of different ages? For some, certain drawings might be easier to identify, while for others, they could be more challenging. As a result, both the chance agreement and observed agreement may vary across different pairs of raters.
This is where Cohen’s Kappa begins to reveal its limitations. While the method works well when the same individuals rate the same items repeatedly, it struggles in more complex situations involving multiple pairs of raters and various items to be rated—what we refer to as ‘nested group scenarios.’
To address this issue, the author developed a new approach. Instead of using the traditional method of combining all the data into a single measure, this new approach considers each pair of raters and each subject they assess individually. This allows for a more accurate and flexible measure of reliability.
This new approach utilizes a mixed model that incorporates both fixed effects and random effects, tailored to each pair of raters based on their level of disagreement (which is calculated as 1 minus their level of agreement) and the probability of agreement by chance. By analyzing this relationship, the model provides a more nuanced understanding of the reliability of assessments. This method is particularly effective in complex, nested scenarios, where traditional methods like Cohen’s Kappa may fall short. By considering the specific characteristics of each pair of raters and the subjects they assess, this approach offers a more accurate and flexible measure of reliability
More about the method can be found in the video, which is a short tutorial that demonstrates the motivation behind the paper, as well as in the paper published here.